Overcoming AI Implementation Challenges in Enterprise Environments
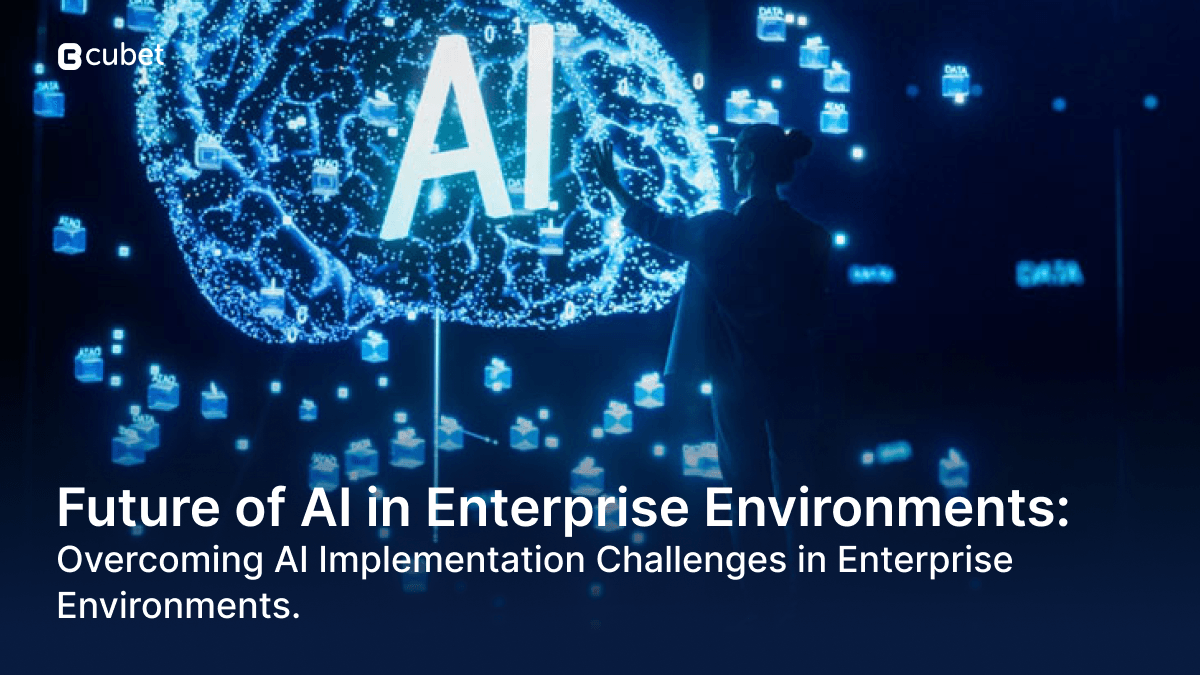
Companies adopting AI are 1.5 times more likely to outperform their peers. This advantage translates to higher revenue growth and profitability. AI's rise in enterprises has greatly improved results, showing how AI drives business success.
However, businesses encounter several hurdles in adopting AI—from needing more skills to dealing with poor data quality and infrastructure limitations. High costs and the need for proper infrastructure can also slow down how quickly companies can effectively adopt AI.
Leaders must manage AI technology while addressing regulatory and ethical concerns. Companies need a solid plan tackling technical and human-side issues to use AI most effectively.
This blog will dive into these challenges and offer actionable advice to overcome these challenges.
Key Challenges in AI Implementation
Everything has its limitations, and so does AI. Here are the main challenges companies face while implementing AI.
1. Identifying the Right Data
The quality and relevance of data have a direct impact on how accurate and reliable AI models will be. Identifying and managing the right data is still a significant challenge for enterprises.
Inconsistent, incomplete, or biased data can harm AI performance. In addition, bias in data can cause major issues, such as wasted resources and poor AI performance. For example, 37% of respondents cited wasted marketing budgets and 35% noted inaccurate targeting due to inadequate data.
Solution:
- Data management: You should use data cleansing techniques to remove duplicates, handle missing values, and standardize data formats. Also, establish protocols for ongoing data collection. This will help ensure you have the latest and most relevant data.
- Addressing the bias: Check your data for bias and use methods like re-sampling or fairness-aware algorithms to keep it accurate. This helps avoid costly mistakes and improves customer experiences.
2. Data Security and Privacy Risks
AI uses large amounts of data to train machine learning models. This comes with data storage and security issues for businesses. To get the most out of AI, you must tackle this issue.
AI advancement has made data security a rising global challenge. Data breaches can lead to severe financial penalties. For example, Equifax had to pay about $650 million to settle claims from a data breach. Yet, issues with AI are not limited to data theft. Companies or individuals can train AI for harmful purposes, like cyber attack bots. Such problems can cause a significant loss of customer trust and involve legal matters.
Solution:
- Implementing robust security measures: Encryption, authentication, and security audits are useful to avoid unauthorized access, protect sensitive data, and ensure data security.
- Managing sensitive information in AI applications: Companies can use data anonymization to protect identities. This ensures privacy without sacrificing the analytical power of AI systems.
3. Infrastructure Limitations
Traditional IT setups serve general-purpose computing needs. They don’t meet the specific needs of AI systems. This includes handling extensive data, using powerful computing, and having scalable storage. Many IT companies lack AI architecture, so upgrading is crucial to leverage AI.
Solution
- Upgrading legacy systems: To implement AI, you must make your system compatible with modern technologies. For adaptable systems, this strategy is the most cost-effective. It will ensure that your AI models get deployed and the existing workflow isn’t disrupted.
- Need for high-performance computing resources: To accomplish AI tasks, large learning models need high computational power. Resources such as GPUs and TPUs can significantly improve the efficiency and speed of AI processes.
- Cloud versus on-premise solutions: Consider the following factors before choosing:
Factor | Cloud-Based Solutions | On-Premises Solutions |
---|---|---|
Flexibility | High; easily scalable | Limited; scaling requires physical upgrades |
Cost | Lower upfront; pay-as-you-go | Higher upfront; ongoing maintenance costs |
Data Security | Managed by the provider; varies | Greater control and customization |
4. Integration With Existing Systems
Integrating new AI solutions with your established workflow takes work. You can face issues at both the application and data level. Incompatible software versions and data formats can disrupt your existing workflow.
Solution:
- Select the right AI vendors: Choosing the right AI vendors can prevent many issues. They manage data quality, storage, performance, and security. Experienced vendors like Cubet understand these challenges and provide effective solutions, reducing implementation risks.
- Ensuring compatibility with current technologies: To scale a business, updating your system with new technology is essential. AI works better with modern tech stacks. However, integrating AI requires a thorough assessment of APIs, data formats, and integration protocols. A solid integration strategy prevents disruptions. It ensures that AI applications improve business operations.
5. Complexity of Algorithms
AI algorithms are much more complex than what people think they are. To develop, understand, and maintain them, you need expertise. Due to the increase in demand and skill shortage, it can slow your implementation and even increase costs. This might pose a significant barrier to AI adoption.
Solution:
- Understanding and developing advanced AI algorithms: Developing AI algorithms requires a deep understanding of modern AI technologies. Enterprises should consider cost-effective methods like training their team to become AI-ready.
- Importance of explainability in AI decision-making: Explainable AI is a responsible approach to developing and integrating AI with their systems. It describes the impact and bias that your AI model can create. It helps you understand model accuracy, fairness, transparency, and outcomes in AI-powered decision-making.
6. Training and Retraining AI Models
Data around the world keeps on changing and updating. Thus, AI models trained on large amounts of data need constant training and retraining. However, training AI models requires correct, complete, and relevant data. Efficient training requires significant computational power. It is essential to design the model to be fair and unbiased. Regularly updating and monitoring models helps catch and fix issues as data patterns change.
Solution:
- Continuous learning and adaptation: Continuous learning involves incremental and lifelong learning. Both approaches enhance accuracy and improve model performance. Design AI systems to continuously learn from new data and acquire new knowledge and skills throughout their operational lifespan.
- Role of AI experts in model development: AI experts develop, train, and maintain AI models. They ensure teams design models correctly, train them on relevant data, and align them with business objectives.
7. Cost Factors
The cost of AI implementation is a matter of concern for many enterprises. A global survey by AI Business found that small businesses often struggle with budget constraints for AI adoption. Implementing AI for small and medium-sized businesses usually becomes a barrier because it involves upfront investments. Acquiring large datasets, training models, and computational resources requires significant costs.
Solution:
- Cost-effective strategies for AI deployment: Enterprises can explore cost-effective strategies like using open-source tools and cloud-based services. This allows for a gradual approach to AI, starting small and scaling up as needed.
Strategies for Overcoming Challenges
Overcoming AI challenges requires a multifaceted approach addressing technical and ethical concerns. Consider the following ways to overcome AI implementation challenges.
1. Building a Strong Data Governance Framework
Data governance consists of the practices and processes for formally managing enterprise data. Its importance grew with the rise in data breaches and theft.
To achieve this, enterprises should focus on two key areas:
- Establishing data quality standards: Enterprises should define guidelines and rules for data management. It is useful for data quality, security, and compliance.
- Implementing data management practices: Data integrity is of utmost importance for enterprises. Ensure responsible data use and conduct regular data audits and validation processes. The practices should include:
- Data Cleansing
- Standardization & Documentation
2. Investing in Infrastructure
We’ve seen how infrastructure limitations can impact performance. This highlights the need to invest in the right infrastructure. Is your enterprise AI-ready and has the correct infrastructure?
- Upgrading IT systems for AI readiness: Assess your IT infrastructure to identify areas needing further assessment and upgrades, such as:
- Hardware, Software & Cloud Capabilities.
- High-Performance Computing Resources.
- Scalable Storage Solutions
- Advanced AI development tools.
- Exploring cloud-based AI solutions: There are several advantages of using cloud-based AI solutions:
- Flexible and Scalable
- Cost-effective
- Usage of Latest AI tools
3. Partnering With Experts
AI experts can equip your enterprise with valuable insights and expertise.
- Collaborating with AI consultants and vendors: Partnering with experienced AI consultants and vendors can streamline implementation. Experts bring knowledge of best practices, tools, and strategies that help enterprises overcome challenges to achieve their AI goals.
- Training and upskilling employees: Enterprises should train employees in AI technologies, data science, and machine learning. This equips them with the skills to develop, deploy, and maintain AI solutions.
Future Directions in AI Implementation
The future of AI implementation is poised for transformative advancements across industries. Let’s explore the trends in detail.
Emerging Trends in AI Technology
AI will expand into new domains, from personalized healthcare and smart cities to more sophisticated cybersecurity measures.
- AI in Healthcare: AI supports drug discovery, disease diagnosis, and personalized treatment plans. It also has revolutionized patient care.
- AI in Finance: In finance, AI actively detects fraud, drives algorithmic trading, and assesses risk.
- AI's Impact on Chatbots: Due to conversational AI and techniques like fine-tuning, AI-powered chatbots respond to humans more naturally and accurately than ever.
Potential for AI To Transform Enterprise Operations
AI is all set to transform enterprise ops in the following ways:
- Power of Automation: AI in enterprises automates several repetitive processes. It also powers and automates the Data Governance process.
- Personalized Customer Support: AI-powered tools provide tailored assistance.
- ChatGPT-enabled AI chatbots: They are transforming how businesses interact online. Companies like Cubet are leading this revolution with their advanced solutions.
- Enhanced Decision Making: AI processes real-time data from multiple sources. It provides insights that help businesses make informed choices.
- Predictive Maintenance: AI detects equipment issues before they become problems. This allows companies to schedule repairs before breakdowns occur, minimizing operational disruptions. It reduces downtime and saves costs.
- Advanced Analytics: AI models analyze customer behavior and preferences. It helps businesses understand trends and make better decisions.
Importance of Adapting to Evolving AI Landscapes
As AI grows, various sectors can enhance efficiency and innovation by applying best practices. Here are some key practices:
- Embrace & train for AI-first world: Understand how AI will help your business grow. Train your employees to stay ahead of the competition.
- Hybrid Approach: Experiment using AI and human combination to perform tasks to see which tasks now need less time and resources than before.
- Leverage Data & Use Ethical AI: Use data to develop AI algorithms to create personalized customer services or products.
Bottom Line
AI implementation can be overwhelming. Companies face several challenges, from data issues to security concerns. These problems make them hesitant to invest in AI-driven initiatives. But in today’s fast-paced world, using AI can benefit your business. It helps you innovate and work more efficiently, leading to greater profitability.
Our team of AI experts at Cubet can help you:
- Prepare your data
- Choose the right AI models
- Integrate AI with your existing system
- Ensure ethical AI practices
- Provide ongoing support
As Jeff Bezos said, “AI is going to change the world. It's going to change every business on the planet. ” Let AI help automate and optimize several processes for you. This will help your organization scale and give you a competitive advantage.