Revolutionizing Medical Diagnostics: Early Disease Detection Gets a Boost from AI and ML
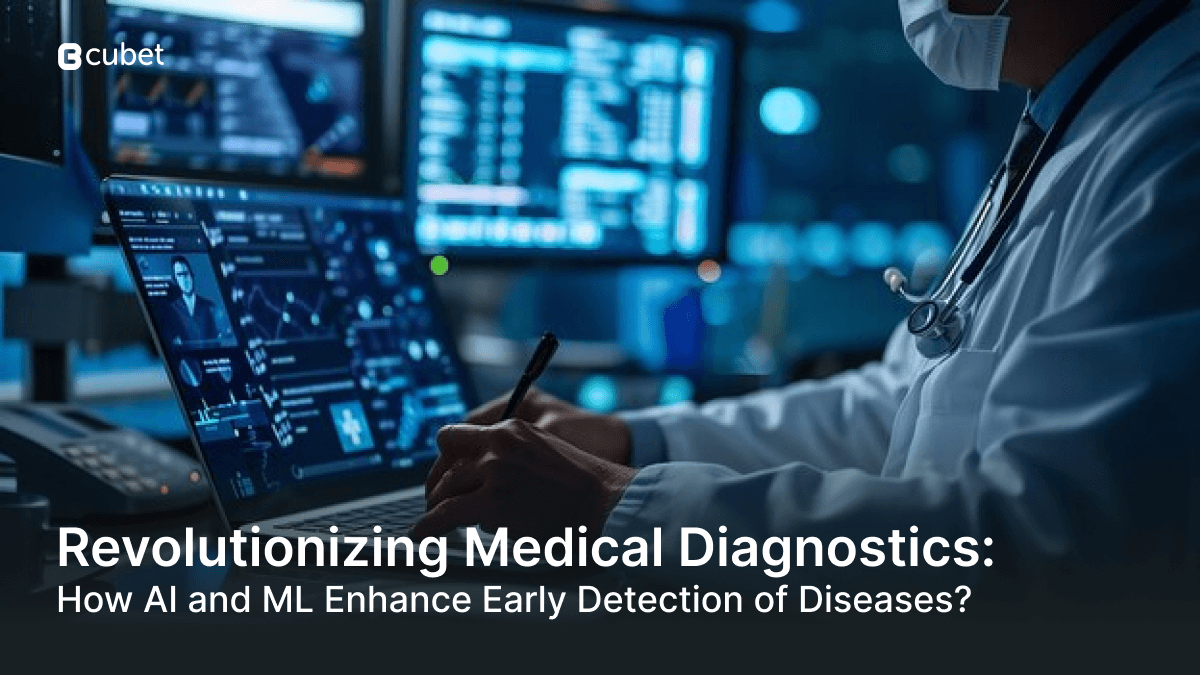
Early disease detection is crucial for successful treatment and better outcomes. But with rising stakes, our tools are more advanced than ever. Artificial intelligence (AI) and Machine Learning (ML) are revolutionizing disease detection in healthcare, often identifying issues before symptoms appear. These technologies improve diagnosis accuracy, enable personalized treatments, save lives, and cut healthcare costs.
This blog looks at how artificial intelligence and machine learning help find diseases early and what that means for the future of healthcare.
What AI and ML Are Doing for Your Medical Diagnostics?
In healthcare, AI refers to the use of computer systems to perform tasks that typically require human intelligence, such as interpreting medical data or making decisions. ML is a subset of AI. It involves algorithms that learn from data to make predictions or classifications.
The Key to Sharper and Faster Diagnostics
AI and ML algorithms go through tons of medical images to detect patterns and abnormalities that doctors might overlook. This makes diagnoses more accurate and speeds up the process. The result: earlier treatment and better results for patients. For instance, ML algorithms for detecting lung cancer have shown 77.8% to a perfect 100% accuracy!
Why AI Needs Strong Data and Computing Power?
AI and ML in health, fitness, and medical diagnostics rely on vast datasets, like medical records and images, for accurate predictions. Advanced computing power enables quick, complex calculations and real-time data processing. This improves system performance and diagnostic precision.
Early Detection Hacks: Techniques That Work
Early disease detection is changing healthcare. With new, advanced methods, we can catch illnesses right from the start. Here's how:
ML Algorithms
ML algorithms are making more accurate and efficient diagnoses. Here are a few different types commonly used in medical diagnostics:
1. Logistic Regression (LR)
LR algorithm helps with binary classification. For example, it can figure out if a patient has a certain disease based on different factors. It calculates the odds and sorts the data accordingly.
2. Support Vector Machines (SVM)
SVMs are like drawing a line between two groups of data. This "line" helps classify the data. In healthcare, SVMs are used to categorize patient data to diagnose diseases, like identifying tumors from MRI scans.
3. Decision Trees
Decision tree algorithms learn from data. Then, they use simple decision rules to make predictions. This helps figure out aspects like risk levels and potential disease outcomes, making them useful for assessing health risks and forecasting results.
4. Random Forests
Random Forests is like having a team of decision trees working together to make better predictions and avoid mistakes. This method combines many trees to improve accuracy and reduce overfitting. It's popular in diagnostics because it's both strong and reliable.
5. Neural Networks
Convolutional Neural Networks (CNNs) and Recurrent Neural Networks (RNNs) are key players in handling complex data. CNNs are great at analyzing images, like X-rays or MRIs. On the other hand, RNNs are perfect for tracking data over time, such as monitoring patient records.
6. Naive Bayes
This algorithm uses probabilities to make predictions. It’s handy for diagnosing diseases. It looks at symptoms and figures out the likelihood of different conditions.
7. K-Nearest Neighbors (KNN)
KNN is a super simple, instance-based algorithm. To classify data, it looks at the closest examples it’s trained on. It’s great for identifying disease similarities and grouping patient profiles.
Applications of ML Algorithms
ML algorithms have diverse applications in medical diagnostics:
- CNNs analyze medical images like X-rays and MRIs to spot abnormalities precisely.
- ML algorithms read genetic sequences to predict hereditary diseases and personalize treatments.
- Algorithms examine patient data and history to predict disease onset or progression for early intervention.
- ML scans EHRs to uncover patterns for predicting outcomes and recommending personalized care, improving patient management.
Deep Learning and Computer Vision
Deep learning and computer vision excel in analyzing X-rays and MRIs, detecting tumors or fractures with remarkable accuracy, often spotting issues humans might overlook. As a result:
- Diagnostic accuracy improves.
- Disease detection happens earlier.
- Patient outcomes become better.
Case Study 1: The Impact of Transfer Learning on CNN Performance and Accuracy
Transfer learning has really boosted how well Convolutional Neural Networks work in dermatology, even with the tricky differences in skin color. Here’s how:
- Initially, differences in skin tones led to missed lesion detections.
- Transfer learning overcame limited data, boosting model accuracy to 92%, highlighting its potential in healthcare diagnostics.
Case Study 2: Improving Data Integration and Predictive Capabilities
Biomedical research collects complex data from various sources, like health records and medical images, often lacking proper labeling.
- Deep learning initially excelled at managing diverse healthcare data, showcasing its potential early on.
- Current methods need improved data integration, clearer interpretation, stronger security, and better handling of time-based data for clinical application.
- It could drive future predictive healthcare systems, processing billions of records for comprehensive patient insights.
- It supports targeted and exploratory research, integrating insights from varied data types.
Natural Language Processing (NLP)
Breaking Down Clinical Notes and Patient Records with NLP
Natural Language Processing uses advanced text mining to handle unstructured data. It pulls out key details from free-text notes, sums up patient histories, and identifies symptoms or conditions. This helps with quick access to critical patient info, improving decision-making and workflow efficiency.
Extracting Insights for Evidence-Based Medicine
NLP algorithms analyze research, clinical trials, and patient records to identify trends and correlations, aiding in treatment guidelines, personalized plans, and keeping doctors updated on medical breakthroughs.
From Theory to Practice: AI's Real-World Diagnostic Uses
AI brings many new helpful solutions to the table for all sorts of healthcare applications:
Improving Radiology and Pathology
In radiology and pathology, AI improves image analysis with precision. CNN algorithms analyze X-rays and MRIs to detect abnormalities, classify lesions, and improve diagnostic accuracy. AI in pathology identifies cancer cells and reduces errors.
AI in Wearable Devices for Continuous Health Monitoring
AI wearables provide continuous health monitoring and real-time insights into multiple physiological parameters, like:
- heart rate
- activity levels
- blood sugar
After all, these devices use sensors and smart algorithms to detect early health signs. This way, they provide personalized advice from your wrist or body.
Predictive Analytics for Acute Conditions
AI-powered predictive analytics identify acute conditions like kidney injury. It analyzes patient data and trends. This allows doctors to start early with treatment and prevention, improving outcomes and reducing complications.
AI and ML: The Benefits of Early Detection
AI and ML offer many benefits in the timely detection of diseases. Here's how:
Improved Diagnostic Speed and Accuracy
AI and ML assess complex data like medical images, genetic info, and patient records to detect patterns that doctors might miss. For instance, ML models can analyze X-rays or MRIs, giving accurate results and speeding up diagnoses for cancer or brain disorders.
Reduction in Healthcare Costs through Early Intervention
AI and ML ease catching diseases early, which helps avoid expensive treatments later on. This saves a lot of money compared to dealing with advanced-stage conditions. Plus, AI and ML can stop complications from worsening. So, the need for costly emergency visits or long hospital stays decreases.
Alleviation of Workload for Healthcare Professionals
AI and ML ease healthcare professionals' workload. They handle routine tasks like image analysis and data processing. This allows more patient interaction, improves job satisfaction, and prevents burnout.
What to Watch Out for: Challenges in AI Diagnostics
Despite AI and ML advancements for healthcare, there are still some big challenges we need to think about.
Data Privacy and Ethical Concerns
AI systems need access to sensitive patient data. This raises concerns about data security and consent. If medical data is breached, misuse is possible. Plus, ethical issues arise from AI decision-making and potential algorithmic biases. So, powerful data protection and transparent AI practices are a must.
Integration of AI Systems into Existing Healthcare Frameworks
Many healthcare facilities still use legacy systems that struggle with modern AI tech. Integrating AI with existing EHRs is tough. It needs perfect interoperability for smooth data flow. Plus, staff need training to use these new tools.
Need for Continuous Monitoring and Validation of AI Tools
AI algorithms may get less effective with new or changing medical data. A model trained on one group might underperform with another. Regular updates and checks help ensure accuracy and reliability with evolving patient data.
AI Applications in Healthcare Departments
The vast potential of AI makes it useful for a variety of departments and disease diagnosis.
Cardiovascular Diseases
AI helps doctors catch heart issues before any symptoms come up. Whether it’s predicting heart attacks or analyzing electrocardiograms (ECGs), AI can spot irregularities that doctors might miss, making them notice the disease before symptoms get serious.
Neurological Disorders
AI helps catch brain disorders like Alzheimer’s and Parkinson’s way earlier than traditional ways. It scans the brain to identify subtle changes. Plus, it keeps tabs on how patients respond to meds, giving doctors better info to tweak treatments.
Oncology
AI is amazing at spotting tumors in imaging scans. It can even recommend personalized treatment plans tailored to a person’s unique genetic makeup. Plus, AI can predict how likely cancer is to spread. This way, doctors can jump in and treat the disease early.
Diabetes Management
AI helps predict blood sugar fluctuations based on food, activity, and medication. To help patients stay on top of their conditions, some AI-powered apps can suggest:
- meal plans
- exercise routines
- insulin doses
Dermatology
AI checks skin spots and moles to detect early signs of skin cancer. It can figure out which moles look dangerous just from images. This gives dermatologists a faster and more accurate way to make diagnoses.
Ophthalmology
AI in eye care is used for diagnosing conditions like diabetic retinopathy and glaucoma. When it analyzes retinal scans, it spots early signs of these diseases. This allows for early treatment.
Mental Health
AI-powered chatbots and apps step in to help people dealing with anxiety, depression, and other mental health health issues. These tools can track the patient's mood, suggest therapy exercises, and give you a safe, non-judgmental space to share what their feeling.
Conclusion
Staying current with AI and ML research can help with better medical diagnostics. Improving these technologies will make diagnoses more accurate, create personalized treatments, and expand AI use. This will transform healthcare and improve patient care.
Cubet excels at AI services like Computer Vision, ML Model Development, and Predictive Analysis — key players in transforming healthcare diagnostics. Want to see how Cubet’s skills can upgrade your medical diagnostics? Reach out to us today to find out more!