Can AI Governance Overcome Its Biggest Challenges as AI Evolves?
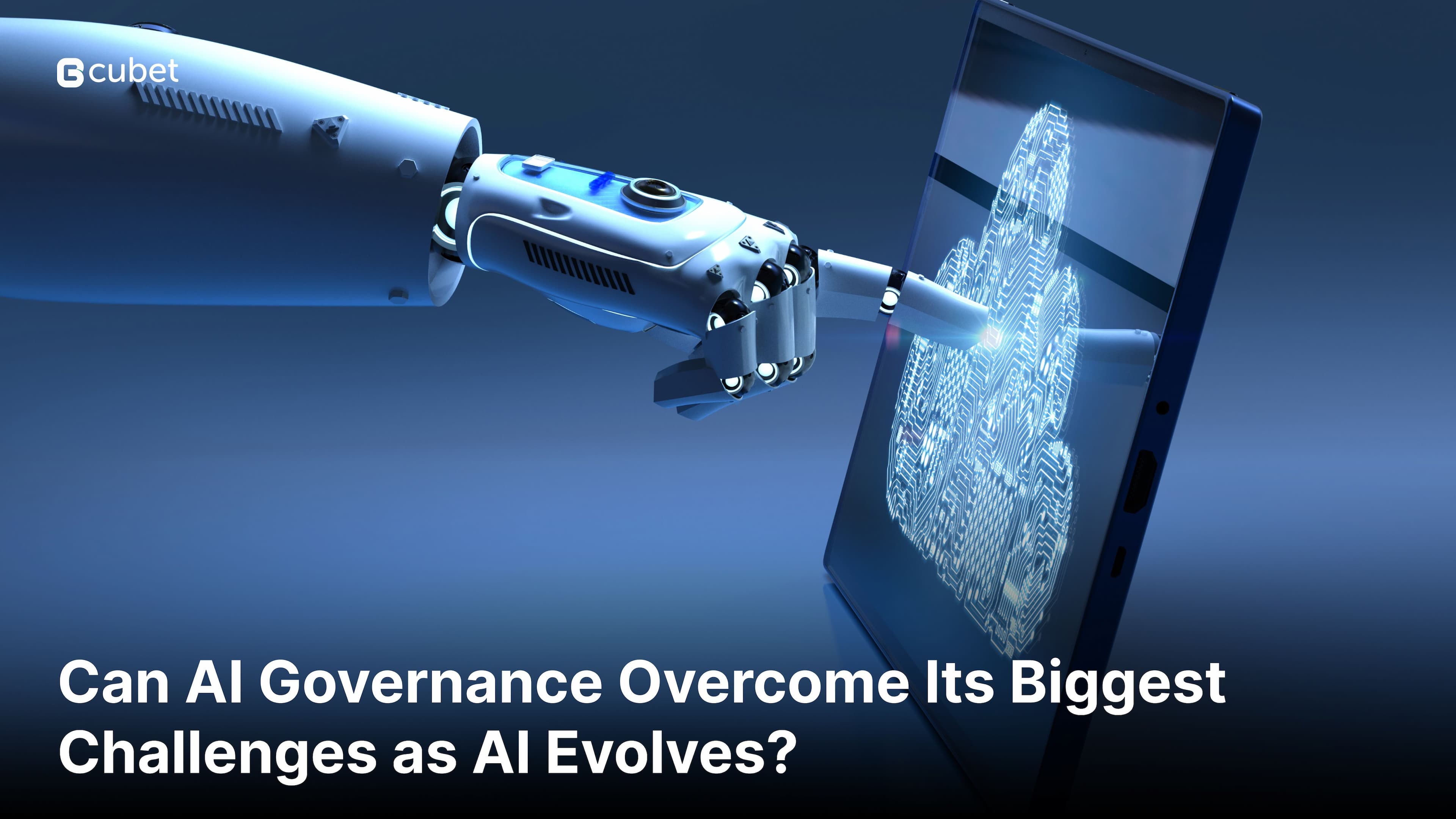
As AI systems become deeply embedded in industries from healthcare diagnostics to autonomous vehicles the conversation isn’t just about what AI can do, but what it should do. The breakneck speed of AI innovation has outpaced our ability to manage its risks, leaving gaps that could undermine trust, safety, and fairness. This is where AI governance steps in: the framework that ensures AI is developed and deployed responsibly. But building this framework isn’t straightforward. Let’s unpack why governance matters, the hurdles we face, and how we can tackle them.
What Exactly Is AI Governance?
AI governance is the set of policies, practices, and ethical guidelines that steer the development and use of AI systems. Think of it as the “rules of the road” for AI ensuring these technologies align with societal values, comply with regulations, and avoid harm. It’s not about oppressing innovation; it’s about creating guardrails so innovation advances safely.
But here’s the catch: AI isn’t like traditional software. It learns, adapts, and sometimes acts in ways even its creators don’t fully predict. Governing such a dynamic force requires balancing flexibility with accountability, a tightrope walk that’s easier said than done.
Why Governance Can’t Wait?
Five years ago, AI governance felt like a niche concern. Today, it’s urgent. High-profile missteps—like biased hiring algorithms or chatbots expelling harmful content—have made headlines, eroding public trust. Meanwhile, governments are scrambling to draft regulations (e.g., the EU’s AI Act, U.S. Executive Orders on AI), and companies face mounting pressure to prove their AI isn’t just powerful, but ethical.
As Andrew Ng, a leading AI researcher, puts it:
“AI is the new electricity. But unlike electricity, AI has the potential to impact human rights, privacy, and fairness at scale.”
The stakes are too high to treat governance as an afterthought.
Five Big Challenges in AI Governance
One of the key debates in AI governance is the tension between promoting open AI innovation and imposing restrictive, costly licensing regimes. Excessive regulation risks stifling creativity, consolidating power among a few large players, and hindering global collaboration. However, a lack of accountability is equally dangerous. No AI developer or deploying organization should be immune to consequences for misuse—whether it’s enabling discrimination, spreading harmful content, or making unethical decisions. The solution lies in frameworks that encourage innovation while mandating transparency and responsibility. “Blaming the algorithm” cannot absolve humans of accountability.
1. Who Decides What’s “Fair”?
AI systems often mirror the biases in their training data. A resume-screening tool might favor candidates from certain universities, or a facial recognition system could misidentify people of color. Fixing this isn’t just a technical problem—it’s a human one.
Who defines fairness? A developer in Silicon Valley might have a different perspective than a regulator in Brussels or a farmer in Kenya. Even with the best intentions, bias can creep in through ambiguous metrics. For example, an AI model optimizing for “employee productivity” might unfairly penalize remote workers with caregiving responsibilities.
2. Navigating a Patchwork of Laws
The EU’s AI Act classifies AI systems by risk levels, banning some (like social scoring) outright. The U.S. leans on sector-specific rules, like healthcare or finance regulations. China mandates algorithmic transparency. This patchwork creates chaos for global companies.
For instance, an AI model trained primarily on Al Jazeera news data might report differently on the Israel-Palestine conflict compared to one trained on CNN’s coverage. This variability complicates global governance—how can regulators ensure “neutrality” when the training data itself reflects regional or editorial biases? Compliance becomes complex—costly, slow, and prone to missteps.
3. The Black Box Problem: “Why Did the AI Do That?”
Many AI models, especially deep learning systems, operate as “black boxes.” They make decisions without explaining how. This lack of transparency is a governance nightmare. If a loan application is denied by an AI, the applicant deserves to know why. If a self-driving car goes off course unexpectedly, engineers need to diagnose the flaw.
Explainable AI (XAI) tools aim to solve this, but they’re still evolving. For now, organizations often face a trade-off: simpler models that are easier to explain vs. complex ones that perform better but are opaque.
4. Security Risks: When AI Becomes the Attack Surface
AI systems are targets for hackers. Adversarial attacks can trick image recognition with subtly altered inputs (e.g., a stop sign misclassified as a speed limit sign). Data poisoning—corrupting training data—can manipulate outcomes. Even model theft is a risk: stealing proprietary algorithms to replicate or sabotage them.
Traditional cybersecurity measures aren’t enough. AI governance must include safeguards specific to these vulnerabilities, like thorough testing for adversarial security and secure data pipelines.
Navigating the Maze
So, how do we tackle these challenges? Here’s where companies can start:
- Embed Ethics Early: Integrate ethical reviews into the AI development lifecycle. Tools like fairness audits and impact assessments should be as routine as code testing.
- Collaborate Across Borders: Work with industry peers, regulators, and civil society to shape global standards. Initiatives like the Partnership on AI show the power of collective action.
- Invest in Explainability: Prioritize R&D in XAI tools and adopt models that balance performance with transparency.
- Build Adaptive Governance: Treat policies as living documents. Regularly update risk frameworks to reflect new threats and technologies.
- Train Teams (and Leaders): Governance isn’t just a compliance task. Engineers need ethics training; executives must understand AI risks at a strategic level.
The Road Ahead
The future of AI governance won’t be static. We’ll see more real-time monitoring tools, AI systems that audit themselves for bias, and global “sandboxes” for testing innovations safely. Regulation will likely merge around core principles (e.g., transparency, accountability) while allowing flexibility in implementation.
But the biggest shift will be cultural. Governance can’t just be a checklist—it needs to be a mindset. As Brad Smith, Microsoft’s Vice Chair, notes:
“The tech that empowers us also obligates us. For AI, that obligation is to earn trust through accountability.”