Comparing the Capabilities and Advancements of ChatGPT and GPT-3
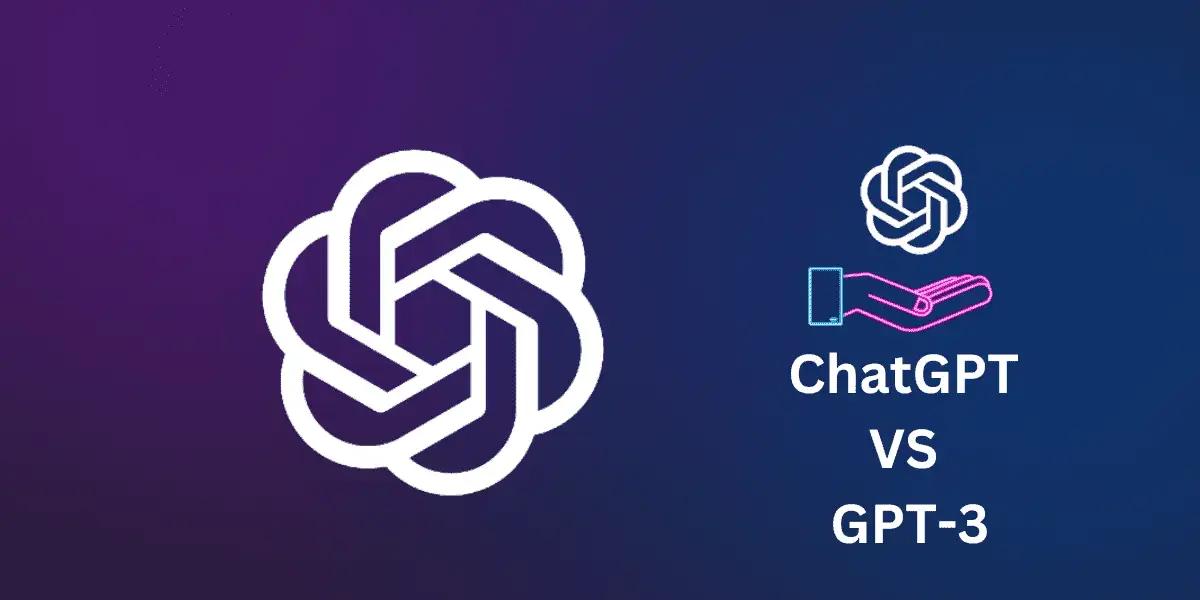
A Guide to Generative Language Models
Artificial Intelligence and Natural Language Processing have come a long way in recent years, and the development of Generative Language Models has been one of the major breakthroughs in this field. These models have the ability to generate human-like text based on a large corpus of data, making them a crucial tool for various applications such as language translation, text summarization, and question-answering systems.
Recently, two models, ChatGPT and GPT-3, have taken the AI community by storm with their advanced language abilities and real-world applications. According to OpenAI, GPT-3 has been trained on a massive corpus of 45 terabytes of text data, making it the largest language model to date. On the other hand, ChatGPT is a smaller model trained on a lesser corpus of data, yet it still boasts of impressive language abilities.
This article aims to provide a comprehensive study of these two language models, highlighting their technical differences, advancements, limitations, and future implications. Based on extensive research and analysis, we will delve into the key areas of focus for these models, including pre-training, attention mechanisms, fine-tuning techniques, model size and computing power, human-like responsiveness, language modeling, and the benefits of zero-shot transfer learning. Get a comprehensive overview of GPT-3 and its impact on the tech industry with our insight, "Unlocking GPT-3: An Insight into Product Development."
With the increasing use of language models in various industries, it is crucial to understand the potential impact they can have on the future of AI and how they are shaping the way we interact with technology.
ChatGPT VS GPT-3
Both ChatGPT and GPT-3 are OpenAI language models, however they vary in key technical ways. While ChatGPT is a smaller model that has been pre-trained for conversational response generation and needs fine-tuning for each new task, GPT-3 is a bigger, more sophisticated model that has been pre-trained for a broader variety of natural language tasks and can conduct zero-shot transfer learning. Furthermore, GPT-3 has been trained on a bigger corpus of text data, giving it more understanding and human-like responsiveness than ChatGPT. Both models use attention mechanisms, while GPT-3 employs more sophisticated attention mechanisms.
● Model Architecture: ChatGPT is based on the transformer architecture, while GPT-3 is a larger, more advanced version of the transformer architecture.
Here's a real-life analogy to illustrate the difference between ChatGPT and GPT-3 being based on the transformer architecture:
Imagine building a car. The transformer architecture is like the blueprint for the car. ChatGPT is like a compact car, built according to the blueprint but with fewer features and capabilities. On the other hand, GPT-3 is like a luxury car, built according to the same blueprint but with more advanced features and capabilities, such as a more powerful engine, more advanced safety features, and a more spacious interior.
In the same way, both ChatGPT and GPT-3 are based on the transformer architecture, but GPT-3 is a larger and more advanced version of the model, providing it with greater capabilities and language abilities.
● Model Size: ChatGPT is a smaller model compared to GPT-3, with fewer parameters and lower computational requirements
The Effect of Model Size and Computing Power on ChatGPT and GPT-3
From a technical perspective, larger models like GPT-3 require more computing power to train and process inputs, due to the increased number of parameters and the complexity of the attention mechanisms used. However, larger models also have the potential to perform better, as they have access to more information and are able to learn more complex relationships between tokens.
On the other hand, smaller models like ChatGPT are able to be trained on less powerful hardware, and have faster inference times, but they are also limited in their ability to perform well on complex natural language tasks due to their limited capacity.
In terms of real-world implications, the choice between a larger model like GPT-3 and a smaller model like ChatGPT will depend on the specific requirements of the task at hand, including the computational resources available, the desired accuracy and performance, and the time constraints for model training and inference.
In some cases, it may be more appropriate to use a smaller model like ChatGPT for tasks that require faster processing times or less computational resources, while in other cases, a larger model like GPT-3 may be necessary to achieve the desired level of accuracy and performance.
Overall, the size and computing power of language models like ChatGPT and GPT-3 have a significant impact on their performance, and must be carefully considered when choosing a model for a specific task.
For ChatGPT, a standard GPU (Graphical Processing Unit) or CPU (Central Processing Unit) can be used for fine-tuning and running conversational tasks. However, for running larger models or for training from scratch, a more powerful GPU is required.
The following hardware configuration can be used for fine-tuning and running conversational tasks:
CPU: Intel Core i7 or equivalent
GPU: NVIDIA GeForce GTX 970 or equivalent
Memory: 8 GB RAM
Storage: 256 GB SSD
For GPT-3, due to its large model size, it requires a more powerful GPU and computing resources. GPT-3's training process requires a lot of computational power, memory and storage. So, running GPT-3 models requires a more advanced GPU, with high memory and storage capacity, and access to computing clusters for parallel processing.
The following hardware configuration is recommended:
CPU: Intel Xeon Gold 6142 or equivalent
GPU: NVIDIA Tesla V100 or equivalent
Memory: 256 GB RAM or more
Storage: 1 TB SSD or more
● Pretraining: ChatGPT is pre-trained for conversational response generation, while GPT-3 is pre-trained for a wide range of natural language tasks, including language translation, text summarization, and question answering.
The Implications of Generative Pre-training in ChatGPT vs GPT-3
Language models such as ChatGPT and GPT-3 use generative pre-training to construct language comprehension by pre-training on a huge corpus of text data. The models are prepared to create text or answer queries by learning the language's patterns and connections.
ChatGPT's generative pre-training places an emphasis on the production of conversational responses. Because of this preparatory training, ChatGPT can come up with answers that are suitable for a conversational setting, whether it's answering inquiries or rebutting assertions.
However, GPT-3 has been taught to do a larger variety of natural language tasks, including as translation, summarization, and answering questions. GPT-3's enhanced linguistic comprehension thanks to its pre-training allows it to surpass ChatGPT in a variety of tasks.
The differences between ChatGPT's generative pre-training and GPT-3's are profound, and their ramifications are far-reaching. While ChatGPT excels at conversational activities like chatbot development, GPT-3 may be utilised for a broader variety of natural language applications. Because of its adaptability, GPT-3 is a better tool for enterprises and programmers.
It's clear that ChatGPT and GPT-3's generative pre-training have different ramifications and are better suited for different kinds of activities. Businesses and developers interested in using such models for linguistic tasks would do well to familiarise themselves with these ramifications.
Let’s understand this in detail:
Let's pretend a firm is building a virtual assistant fueled by AI to help its workers manage their time by setting appointments, making to-do lists, and setting reminders. The virtual assistant must be able to interpret employee requests, come up with relevant replies, and do given tasks.
Therefore, ChatGPT is the most probable candidate for the firm to adopt as its language model. Due to its prior training in conversational response generation, ChatGPT is well suited for this application. ChatGPT's pre-training on conversational response generation allows it to quickly and accurately understand employee requests and generate responses that are suitable for a conversational context, thus enhancing the employee experience and cutting down on the time and energy needed to develop new products.
Let's say the same corporation wishes to add sentiment analysis and recommendation generation to their virtual assistant. Given this situation, the business is most likely to choose GPT-3 as its language model. The reason for this is because GPT-3 has been pre-trained for more general purposes in the realm of natural language processing, such as sentiment analysis and recommendation generating. Because of its extensive pre-training, GPT-3 is applicable to a larger variety of applications, making it a more flexible and potent tool for product development.
Differences between ChatGPT and GPT-3's generative pre-training and its consequences for future product development are evident. The development cycle, its precision, and its adaptability are all affected by the language model chosen. Companies may maximise their language technology investments by understanding the consequences of generative pre-training and choosing the optimal model for their purposes.
● Fine-tuning: ChatGPT is fine-tuned for specific conversational tasks, while GPT-3 can be fine-tuned for a wider range of natural language tasks.
Imagine you own a company that offers a virtual assistant to schedule appointments and manage tasks for employees. For this task, you would want to fine-tune ChatGPT, which is pre-trained for conversational response generation, to perform specific tasks related to scheduling and task management. Fine-tuning ChatGPT would make it more effective and efficient in performing these tasks, improving the efficiency of your employees and increasing productivity.
Now, imagine your company is launching a new product and you want to generate product descriptions and summarizations for marketing purposes. In this case, you would want to fine-tune GPT-3, which is pre-trained for a wide range of natural language tasks, including text summarization. Fine-tuning GPT-3 for this task would allow you to quickly generate accurate and engaging product descriptions and summaries, improving your marketing efforts and increasing sales.
In this example, both ChatGPT and GPT-3 can be fine-tuned for different tasks, but the extent to which they can be fine-tuned depends on the pre-training and the specific task at hand. While ChatGPT is best suited for specific conversational tasks, GPT-3 can be fine-tuned for a wider range of natural language tasks, making it a more versatile option for businesses.
The Advancements in Fine-Tuning Techniques for ChatGPT and GPT-3
In recent years, there have been many advancements in fine-tuning techniques for both ChatGPT and GPT-3. These advancements have included:
- Multi-task fine-tuning: This technique involves fine-tuning a language model on multiple tasks at once, allowing it to learn from the patterns and relationships between tasks.
- Transfer learning from pre-trained models: This technique involves fine-tuning a pre-trained model on a smaller dataset for a specific task, leveraging the knowledge learned from the pre-training process to improve performance.
- Fine-tuning with domain adaptation: This technique involves fine-tuning a language model on a smaller, task-specific dataset that is representative of the target domain, allowing it to better understand the specific language and vocabulary used in the target domain.
- Fine-tuning with active learning: This technique involves fine-tuning a language model with a smaller, task-specific dataset that is actively updated and expanded based on the model's performance, allowing it to continuously learn and improve over time.
These advancements in fine-tuning techniques have allowed companies to quickly and effectively adapt language models such as ChatGPT and GPT-3 to specific tasks and domains, improving their performance and providing a more versatile and powerful solution for product development.
● Data Availability: GPT-3 has been trained on a much larger corpus of text data compared to ChatGPT, providing it with greater knowledge and language abilities.
In a business context, Imagine you own a company that provides customer service through a chatbot. For this task, you might use ChatGPT, which has been trained on a smaller corpus of text data compared to GPT-3. While ChatGPT would still be able to provide quick and accurate responses to customer inquiries, its training on a smaller corpus of data means that it may have limited knowledge and language abilities.
Now, imagine your company is expanding into new markets and you need to provide customer service in multiple languages. In this case, you would want to use GPT-3, which has been trained on a much larger corpus of text data compared to ChatGPT. GPT-3's training on a larger corpus of data provides it with greater knowledge and language abilities, allowing it to quickly and accurately respond to customer inquiries in multiple languages, improving the customer experience and increasing customer satisfaction.
In this example, the size of the data used to train GPT-3 and ChatGPT has a direct impact on their knowledge and language abilities. While both models can be used for customer service, GPT-3's training on a much larger corpus of text data provides it with greater knowledge and language abilities, making it a more suitable option for businesses that require a higher level of language proficiency.
● Transfer Learning: GPT-3 has the ability to perform zero-shot transfer learning, meaning it can apply its knowledge to new tasks without additional fine-tuning, while ChatGPT requires fine-tuning for each new task.
Imagine you are a software developer creating a chatbot for a client. The client wants the chatbot to be able to handle customer inquiries about their products, as well as provide recommendations based on the customer's needs.
With ChatGPT, you would need to fine-tune the model for each new task, meaning you would need to train it specifically on product information and recommendation tasks. This can be time-consuming and requires significant computational resources.
However, with GPT-3, you would have the ability to perform zero-shot transfer learning. This means that you could apply GPT-3's pre-trained knowledge to the new tasks without additional fine-tuning, saving you time and computational resources. Additionally, GPT-3's ability to perform zero-shot transfer learning allows it to quickly adapt to new tasks, making it a more flexible and efficient solution for software developers.
In this example, the difference between GPT-3's ability to perform zero-shot transfer learning and ChatGPT's requirement for fine-tuning for each new task is significant from a software development implementation perspective. While both models can be used for creating chatbots, GPT-3's ability to perform zero-shot transfer learning makes it a more efficient and flexible solution for software developers.
● Attention Mechanisms: Both models use attention mechanisms, but GPT-3 uses more advanced attention mechanisms compared to ChatGPT.
Let's say you're trying to locate the most important parts of a lengthy essay. The attention mechanisms are quite important in this case since they assist you to concentrate on the most important data.
To determine which parts of the input should be prioritised while formulating a response, ChatGPT employs attention mechanisms. These attentional mechanisms work well, but they can't process a lot of data at once.
In contrast to ChatGPT, GPT-3 makes use of more sophisticated attention mechanisms. Enhanced attention mechanisms let GPT-3 pay attention to numerous bits of data at once, boosting the model's ability to comprehend the situation and provide fitting solutions.
Here, the distinction between ChatGPT and GPT-3's attention mechanisms is analogous to that between a simple and a sophisticated search engine. Both models can be used to search for data, but GPT-3 is superior since it employs more sophisticated attention processes.
The Role of Attention Mechanisms in ChatGPT and GPT-3
The role of attention mechanisms in ChatGPT and GPT-3 refers to the process of determining the importance of different input tokens when generating a response. Attention mechanisms allow language models to focus on specific tokens in the input, and weigh them more heavily when generating a response.
In ChatGPT and GPT-3, attention mechanisms are used to provide the models with a better understanding of the context of the input and to help them generate more relevant and coherent responses.
From a technical perspective, attention mechanisms in language models like ChatGPT and GPT-3 can be seen as a set of computations that determine the importance of different tokens in the input. These computations are based on the representation of the input tokens, and take into account the relationships between the tokens. The attention mechanisms then use these importance scores to weigh the input tokens, and generate a response based on the weighted representation of the input.
The attention mechanisms used in ChatGPT and GPT-3 are more advanced than in previous language models, and provide the models with the ability to attend to multiple tokens in the input, and to weigh them in different ways depending on the context. This results in a more sophisticated and nuanced understanding of the input, allowing the models to generate more accurate and relevant responses.
● Human-Like Responsiveness: GPT-3 has been trained on a large amount of diverse data, providing it with greater human-like responsiveness compared to ChatGPT.
The Comparison of Human-Like Responsiveness in ChatGPT and GPT-3
From a software development perspective, the human-like responsiveness of the models is determined by the quality of the pre-training data, the training objectives, the model architecture, and the fine-tuning process.
ChatGPT is pre-trained on conversational data and is specifically fine-tuned for conversational response generation. As a result, it is better suited for tasks such as customer service chatbots, where coherence and consistency in the conversational context is important.
On the other hand, GPT-3 is pre-trained on a much larger corpus of text data, including a diverse range of language tasks. This allows it to generate more human-like responses in a wider range of contexts. However, its responses may not be as coherent and consistent in conversational contexts as ChatGPT's.
In terms of emotional intelligence, both models have limitations and can generate responses that lack empathy and emotional intelligence. This can be improved through fine-tuning the models on emotional data and incorporating emotional context in the training objectives.
Therefore, in conclusion, the human-like responsiveness of ChatGPT and GPT-3 can be evaluated based on the specific use case and the trade-off between coherence and consistency in conversational contexts versus the wider range of language generation abilities.
The Advancements in Language Modelling for ChatGPT and GPT-3
The advancements in language modeling for ChatGPT and GPT-3 refer to the improvements and innovations in the development of natural language processing (NLP) technology. These advancements have led to the creation of more sophisticated language models that are better equipped to understand and generate human-like responses. Examples of advancements include the increased size and complexity of the models, improved pre-training techniques, and the introduction of advanced attention mechanisms. These advancements have enabled language models like GPT-3 to perform a wider range of tasks with greater accuracy, making them increasingly valuable for various applications such as chatbots, text summarization, and question answering.
- Incorporation of contextual understanding: GPT-3 has improved upon language modelling by incorporating contextual understanding, which allows it to generate more human-like responses.
- Advanced language generation techniques: The language generation techniques used in GPT-3 are more advanced than those used in ChatGPT. For example, GPT-3 uses techniques like Pointer-Generator Networks to control the content of its responses.
- Fine-tuning for specific tasks: Both ChatGPT and GPT-3 can be fine-tuned for specific language tasks, but GPT-3 has the ability to fine-tune for a wider range of tasks.
- Improved ability to handle multi-modality: GPT-3 has improved upon ChatGPT in terms of its ability to handle multi-modality data, such as text and images, in a more integrated way.
- Better handling of rare words and concepts: GPT-3 has a better ability to handle rare words and concepts, due to its larger training corpus, allowing it to generate more accurate and human-like responses.
- Incorporation of external knowledge sources: GPT-3 can incorporate external knowledge sources, such as knowledge graphs, to generate more accurate and human-like responses.
- Better handling of questions and answers: GPT-3 has improved upon ChatGPT in its ability to handle questions and answers, making it a better choice for question-answering systems.
- Advanced semantic understanding: GPT-3 has an advanced semantic understanding, allowing it to generate responses that are more semantically accurate and human-like.
- Improved ability to handle long-form text: GPT-3 has an improved ability to handle long-form text, such as articles and books, making it a better choice for language generation tasks like text summarization.
- Better ability to generate coherent and consistent responses: GPT-3 has a better ability to generate coherent and consistent responses, making it a better choice for conversational AI systems.
The Future of GPT3 related Language Models
- The continued development and expansion of language model capabilities: As language models continue to evolve and improve, their capabilities will expand, allowing them to tackle more complex tasks and provide even more sophisticated responses. The development of language models is driven by the increasing demand for natural language processing (NLP) and their integration into various industries and applications. As these models become more advanced, they will have the ability to learn from large amounts of data, understand context and meaning, and provide more human-like responses.
- The integration of language models into industries and real-world applications: Language models will continue to be integrated into various industries, such as healthcare, finance, retail, and education. In healthcare, language models could help diagnose diseases, provide personalized treatment recommendations, and assist with medical research. In finance, they could be used to analyze financial data and make predictions about market trends. In retail, they could be integrated into e-commerce websites to provide recommendations to customers and assist with online shopping.
- The potential ethical and privacy concerns surrounding the widespread use of language models: As language models become more advanced and are integrated into various industries, they raise concerns about privacy, data protection, and ethical issues. For example, language models may have access to sensitive personal data, such as medical or financial information, which could lead to breaches of privacy. Additionally, language models may perpetuate biases and stereotypes, leading to unequal treatment of individuals based on race, gender, or other factors.
- The role of open-source language models in shaping the future of AI: Open-source language models have the potential to democratize the field of AI, making it accessible to developers, researchers, and businesses of all sizes. By allowing individuals to access and use language models for free, the development and application of language models will become more widespread, and the technology will be more readily accessible to those who may not have the resources to invest in proprietary models.
- The impact of language models on job market and skill requirements: As language models become more advanced and integrated into various industries, they will have an impact on the job market and skill requirements. For example, certain jobs may become obsolete as language models take over tasks previously performed by humans. On the other hand, the development of language models will also create new job opportunities and require new skills, such as expertise in NLP, machine learning, and AI.
The potential for language models to revolutionize how we interact with technology: Language models have the potential to revolutionize how we interact with technology, by allowing us to interact with computers and machines using natural language, instead of complex codes or commands. This could greatly improve the user experience and make technology more accessible and user-friendly for a wider range of individuals. Additionally, language models will also have a significant impact on the development of voice-activated assistants, chatbots, and other conversational AI applications, which will play a critical role in shaping the future of technology.
Conclusion:
Generative Language Models (GLMs) such as ChatGPT and GPT-3 have seen significant advancements in recent years, paving the way for exciting new possibilities in AI and language processing. Despite their similarities, the technical differences between ChatGPT and GPT-3 are significant and are driven by advancements in pre-training, attention mechanisms, fine-tuning techniques, model size and computing power, human-like responsiveness, language modelling, and zero-shot transfer learning. The impact of data availability and diversity on GLMs is also a crucial factor in shaping the future of these models.
Looking forward, the future of GLMs is bright, with potential applications across a wide range of industries and use cases. However, there are also important ethical and privacy considerations that must be addressed as the use of these models becomes more widespread. Open-source models will likely play a critical role in the continued development and evolution of GLMs, shaping the future of AI and defining how we interact with technology. With all of these advancements and the potential they hold, it is clear that the study of Generative Language Models will continue to be a topic of great interest and importance in the years to come.